From her slapdash home office, the RMIT and postdoctoral fellow at the ARC Centre of Excellence in Exciton Science has built an AI algorithm which is not only able to quickly and accurately assess potential PV materials, but can make visible the ways in which sequences of chemical compounds combine to most efficiently convert solar light into electricity.
Published in the journal Nature earlier this month, the model is now available to download for free from Github. For Meftahi, the decision to make the algorithms accessible to the public was a no brainer.
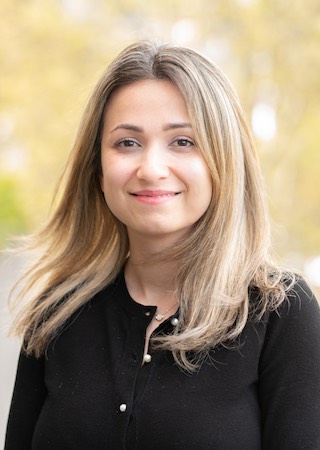
Photo: RMIT
“One of the greatest challenges of using a developed machine learning model for other scientists is the accessibility of the actual models. There are so many models available in the literature but in order to use them, you need to develop the model from scratch and that is not an easy task,” Meftahi told pv magazine Australia.
“I wanted to make sure that anybody by a few clicks can have access to the algorithm that can predict PCE [power conversion efficiency] … if anyone can use our model to make better solar cells, the whole world benefits.”
What makes the model different is that for the first-time signature descriptors, essentially chemically interpretable fragments of the materials, replace the cumbersome parameters requiring quantum mechanical calculations.
“Signature descriptors have been used for many years but mostly in different areas of science such as drug design and development,” Meftahi said. “One of the biggest outcomes of this project was simply proving that signature descriptors can be used to predict properties of organic photovoltaic devices.”
The relatively straightforward parameter switch had not been tried for PV cells before, Meftahi believes, because until recently modules have primarily been made with inorganic compounds like silicon, for which signature descriptors don’t work.
It is only recently that attention has turned to organic photovoltaic (OPV) solar cells, which will be cheaper to make by using printing technologies, as well as being more versatile and easier to deal with at end-of-life. Sorting through the massive volume of potentially suitable chemical compounds that can be synthesised for use as OPV was, however, a substantial barrier.
It is precisely this issue that Meftahi’s model, which was developed with RMIT professor Salvy Russo and Professor Udo Bach’s team at Monash University, goes a way to solve.
How the model works
To develop a solar cell device, there must be electron acceptor and electron donor materials. In the past, experimental scientists have had to manufacture devices, mixing different acceptors and donors one by one in the lab, then measuring the photovoltaic properties. The task, unsurprisingly, requires tremendous time and resources.
Cutting out this real life to-and-fro, the model is able to identify the combination of electron acceptor and donor materials with the highest PCE value. Operating in the universe of 0 and 1s, the model extends to compounds that are not yet in existence. That is, it can pick up fragments and show where efficiency increases and where it decreases.
“It means that material scientists can look at my models, get ideas about the most important fragments and design new and un-synthetized compounds.” These “virtual materials” can then be fed back into the model to have their efficiency predicted.
“If the efficiency is good, they can go to the lab and synthase that virtual material – taking the theory into the practice.”
The new approach could help to significantly speed up the process of designing more efficient solar cells as the need for renewable energy grows.
Since the model was only published a few weeks ago, Meftahi is yet to have received any feedback from industry. “I guess it’s still too soon.”
Fresh as it might be, Meftahi is already working on developing the model with a bigger data set. Currently the accuracy of the model sits between 70% to 80%, with R2 values of 0.72 and 0.83, achieved from data set with 350 data points. Now, the RMIT researcher is working with a new data set consisting of 1500 data points, using the larger and more diverse set to hopefully build an even more accurate predication.
Meftahi is hopeful she will be able to collaborate with her colleagues at the ARC Centre of Excellence in Exciton Science, where a 3D printed system was able to speed up solar cell testing from hours to minutes announced in May is soon to be up and running.
Co-author Professor Dave Winkler of CSIRO’s Data 61, Monash University, La Trobe University, and the University of Nottingham all supported Meftahi in her work, the latter co-creating the BioModeller program that provided the basis for the new, open source model.
This content is protected by copyright and may not be reused. If you want to cooperate with us and would like to reuse some of our content, please contact: editors@pv-magazine.com.
2 comments
By submitting this form you agree to pv magazine using your data for the purposes of publishing your comment.
Your personal data will only be disclosed or otherwise transmitted to third parties for the purposes of spam filtering or if this is necessary for technical maintenance of the website. Any other transfer to third parties will not take place unless this is justified on the basis of applicable data protection regulations or if pv magazine is legally obliged to do so.
You may revoke this consent at any time with effect for the future, in which case your personal data will be deleted immediately. Otherwise, your data will be deleted if pv magazine has processed your request or the purpose of data storage is fulfilled.
Further information on data privacy can be found in our Data Protection Policy.